Running Totals
Running Totals or Cumulative Sums are a powerful way to see not just a trend of data, but also the cumulative results.
For example, if you wanted to monitor your monthly sales, but also make sure you're on track to achieve your annual goal, a running total will sort you out:
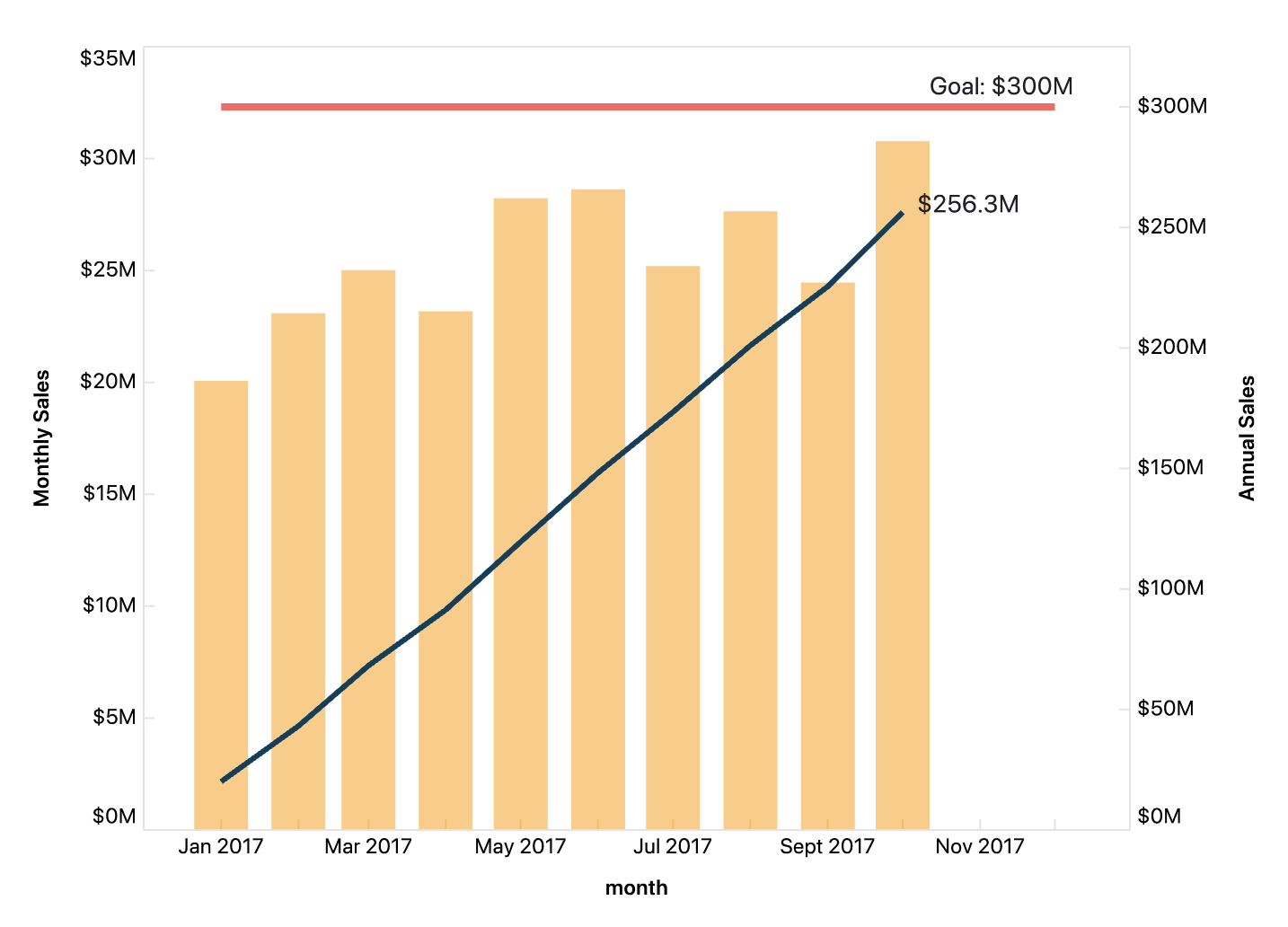
Step-by-Step
Running Totals rely on the use of Window Functions, which you can read more about here.
To find a running total we'll use the SUM
window function:
SUM( <expr1> ) OVER ( [ PARTITION BY <expr2> ] [ ORDER BY <expr3> [ ASC | DESC ] [ <window_frame> ] ] )
Where:
expr1
This is an expression that evaluates to a numeric data type (INTEGER, FLOAT, DECIMAL, etc.).expr2
This is the optional expression to partition by.expr3
This is the optional expression to order by within each partition. (This does not control the order of the entire query output.)Or, in layman's terms:
SUM(column) OVER (PARTITION BY col_to_group_by, ORDER BY column_to_order_by)
In the following example we'll take the running total of hours of Netflix I watched in a given week:
SELECT
DATE_TRUNC('WEEK',START_TIME) WEEK,
DATE_PART('WEEKDAY',START_TIME) DAY,
SUM(DURATION)/(60*60) DAILY_HOURS_WATCHED
FROM
PUBLIC.NETFLIX
WHERE
WEEK = '2018-11-26'
GROUP BY WEEK,DAY
ORDER BY WEEK,DAY
Now if I want to find the running total of hours watched that week, I can do the following:
SUM(DAILY_HOURS_WATCHED) OVER (ORDER BY DAY ASC) RUNNING_TOTAL
SELECT DAY, DAILY_HOURS_WATCHED, SUM(DAILY_HOURS_WATCHED) OVER (ORDER BY DAY ASC) RUNNING_TOTAL FROM ONE_WEEK
We can see our new RUNNING_TOTAL
column increase each day.
This example had no partition, but if we wanted to compare my viewing habits across two different weeks, we would need to PARTITION BY week:
SELECT
DATE_TRUNC('WEEK',START_TIME) WEEK,
DATE_PART('WEEKDAY',START_TIME) DAY,
SUM(DURATION)/(60*60) DAILY_HOURS_WATCHED
FROM
PUBLIC.NETFLIX
WHERE
WEEK IN ('2018-11-19','2018-11-26')
GROUP BY WEEK,DAY
ORDER BY WEEK,DAY
And now to find the running total across the two weeks:
SUM(DAILY_HOURS_WATCHED) OVER(PARTITION BY WEEK ORDER BY DAY ASC) WEEKLY_RUNNING_TOTAL
SELECT
WEEK, DAY, DAILY_HOURS_WATCHED, SUM(DAILY_HOURS_WATCHED) OVER(PARTITION BY WEEK ORDER BY DAY ASC) WEEKLY_RUNNING_TOTAL
FROM
TWO_WEEKS
ORDER BY WEEK,DAY
Now we can see the RUNNING_TOTAL restart for the 2nd week.
How We Built This
This page was built using Count. It combines the best features of a SQL IDE, Data Visualization Tool, and Computational Notebooks. In the Count notebook, each cell acts like a CTE, meaning you can reference any other cell in your queries.
This makes not only for far more readable reports (like this one) but also a much faster and more powerful way to do your analysis, essentially turning your analysis into a connected graph of data frames rather than one-off convoluted queries and CSV files. And with a built-in visualization framework, you won't have to export your data to make your charts. Go from raw data to interactive report in one document.